Geneseeq’s new blood test may detect early-stage pancreatic cancer
Study shows model in development is 'practical, highly accurate'
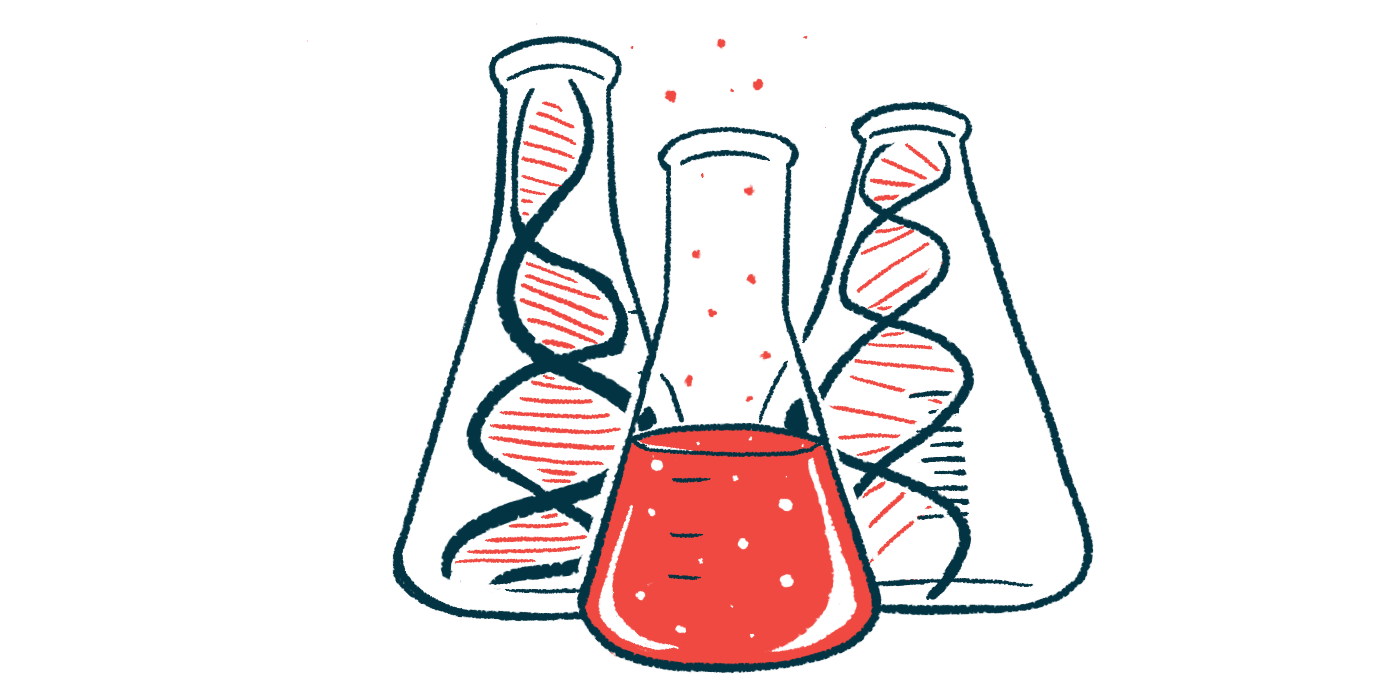
Geneseeq Technology is developing a blood test that uses cell-free DNA (cfDNA) and artificial intelligence (AI) to detect early-stage pancreatic ductal adenocarcinoma — the most common type of pancreatic cancer, which forms in the cells of the pancreas responsible for producing digestive juices — that is often missed by standard screening tests.
In a new study, an AI-based machine learning model looked for patterns in cfDNA — DNA fragments released by cancer cells into circulation — and could accurately distinguish patients with pancreatic ductal adenocarcinoma from healthy individuals. It also differentiated between benign, or noncancerous, lesions and malignant, or cancerous, ones.
“Our cfDNA fragmentomics model offers a practical, highly accurate, and noninvasive option for detecting pancreatic cancer early,” Hua Bao, PhD, vice-president of research and development at Geneseeq, said in a company press release. “It could support earlier identification of at-risk individuals, allowing timely clinical follow-up and potentially improving outcomes.”
The study, “Development and Validation of a Cell-Free DNA Fragmentomics–Based Model for Early Detection of Pancreatic Cancer,” was published in the Journal of Clinical Oncology by a team of researchers in China. Nine of them, including Bao, are employed at Geneseeq.
Eileen M. O’Reilly, MD, the journal’s associate editor, noted that “many novel liquid biomarkers are emerging as tools of potential value to differentiate between benign versus malignant findings in the pancreas.”
Howver, because the study involved only Chinese participants and few with benign lesions, “further validation” is needed, O’Reilly noted.
As a cancer type, pancreatic ductal adenocarcinoma is often diagnosed at an advanced stage, when treatment options are limited and surgery is usually no longer possible. This is largely because the disease rarely causes noticeable symptoms in its early stages. As a result, most patients do not survive beyond five years after diagnosis.
Pancreatic cancer test uses cell-free DNA plus AI
Current screening often fails to detect early signs of pancreatic ductal adenocarcinoma, the researchers noted in citing the need for better diagnostic tests.
Because they are noninvasive, tests such as the Geneseeq one, which look for so-called liquid biomarkers, are becoming more popular for detecting pancreatic cancer. However, these tests can be expensive due to the need for detailed analysis.
Geneseeq’s test looks for patterns in the way cfDNA breaks into smaller fragments, called fragmentomics, using machine learning. Because this type of test does not require as much detailed analysis, it may keep costs lower, making access easier for large numbers of people.
To create a model that could detect pancreatic ductal adenocarcinoma, the researchers collected DNA from blood samples of 166 patients and 167 healthy individuals. In this training data set, the model accurately distinguished patients with pancreatic ductal adenocarcinoma from healthy individuals, the researchers noted.
The model’s accuracy was then validated using data from another 112 patients with pancreatic ductal adenocarcinoma and 111 healthy individuals. This data set also included 67 people with noncancerous pancreatic cysts (lesions).
The model was able to accurately differentiate between patients and healthy individuals. It correctly identified 97.3% of the patients (sensitivity) and correctly ruled out pancreatic cancer in 92.8% of healthy individuals (specificity). It could predict pancreatic cancer even at an early stage. Similar sensitivity and specificity were observed with other data sets.
“Our model effectively identifies [pancreatic ductal adenocarcinoma] at early stages by integrating fragmentomics features with machine learning. This approach holds promise for improving [pancreatic ductal adenocarcinoma] early screening and consequently reduce the mortality rates linked to the condition,” the researchers wrote.
Our approach promises significant potential in reducing [pancreatic ductal adenocarcinoma] mortalities through early intervention and could serve as a breakthrough in oncologic diagnostics.
While the model showed potential clinical value in distinguishing benign from malignant findings, some patients with noncancerous pancreatic cysts had symptoms or underwent surgery, leading to some overlap with patients who had pancreatic cancer.
To further validate the diagnostic specificity of the model in the general population, it was also used to test 20 patients with asymptomatic pancreatic cysts — that is, individuals who showed no symptoms. The model was highly accurate, correctly identifying all as noncancerous, with 100% specificity.
The model also outperformed CA19-9, a commonly used biomarker for detecting pancreatic cancer, particularly in patients with normal bilirubin levels. Bilirubin is a substance produced by the liver that can be elevated in pancreatic cancer.
“Our approach promises significant potential in reducing [pancreatic ductal adenocarcinoma] mortalities through early intervention and could serve as a breakthrough in oncologic diagnostics,” the researchers wrote.