Researchers generate plasma cell dataset to aid myeloma diagnosis
Goal: Improve accuracy, efficiency of diagnostic process in blood diseases
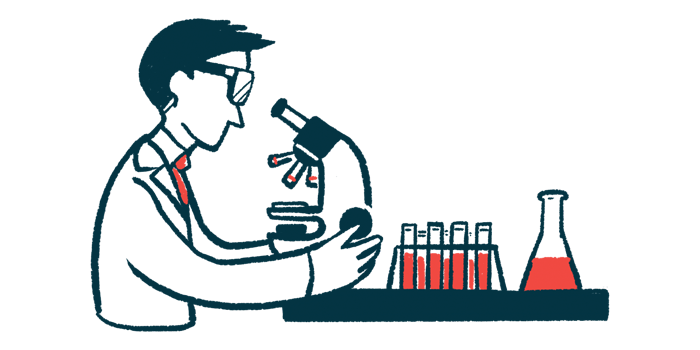
Researchers in Brazil have developed a large dataset of cells taken from the bone marrow of people with multiple myeloma (MM) and other blood disorders that they hope will aid in the correct diagnosis of MM in patients.
According to the team, using this database could help improve the accuracy and efficiency of the diagnostic process — especially in resource-limited areas where there are fewer trained experts.
“Our work improves the practical diagnosis of MM and opens new avenues for advancing the field through improved training, innovative method development, and enhanced accessibility,” the researchers wrote.
In addition to helping doctors learn how to recognize the abnormal cells associated with myeloma, the scientists believe the dataset can be used to develop AI-based algorithms, or those using artificial intelligence, to automatically identify these cells — a time-consuming process usually done manually by a doctor.
The team developed a benchmark algorithm showing the potential of this approach, which the researchers hope will encourage further development of AI-based technologies for diagnosing myeloma.
Their study, “PCMMD: A Novel Dataset of Plasma Cells to Support the Diagnosis of Multiple Myeloma,” was published in the journal Scientific Data.
Current myeloma diagnostic process is time-, labor-intensive
Myeloma is a rare form of blood cancer that develops in plasma cells, a type of immune cell that normally produces the antibodies used by the immune system to fight infections.
The cancer gets its start when a single abnormal plasma cell begins to divide uncontrollably in the bone marrow — the spongy substance found inside bones — and produces large numbers of genetically identical abnormal cells. These cells, called clonal cells, don’t function the way normal plasma cells would.
Instead, the cells overtake the bone marrow and disrupt the growth of healthy blood cells. When myeloma occurs in more than one bone marrow location, which it usually does, it is then called multiple myeloma, or MM for short.
To confirm a myeloma diagnosis, doctors take a biopsy, or a sample of cells, of the bone marrow. If myeloma is present, at least 10% of the cells will be abnormal plasma cells. The analysis is usually done manually by an expert who looks at the tissue under a microscope and counts the cells.
However, this process is labor-intensive, taking significant time and resources. Moreover, there can be inconsistencies in the interpretation of the results — and thus the diagnostic accuracy of the test — depending on the level of expertise of the evaluator. This can be a particular challenge in resource-limited areas where there may be fewer trained experts.
To help overcome these barriers and improve the myeloma diagnostic process, a team led by researchers from the Federal University of Bahia Institute of Computing in Salvador worked to develop a large dataset of more than 5,000 plasma and non-plasma cells. The aim was to create a repository that could be used to help train doctors to identify myeloma.
To generate the dataset, which they called PCMMD — for Plasma Cells for Multiple Myeloma Diagnosis — bone marrow samples were collected from individuals with multiple myeloma or other blood-related diseases who were diagnosed and treated within the Brazilian Public Health System.
Thousands of these cells were visualized with a microscope and photographed with a smartphone camera. Experts in blood disorders, known as hematologists, then manually analyzed the images and labeled all cells as either plasma or non-plasma cells.
Scientists hope to improve myeloma diagnosis in resource-limited areas
In addition to helping doctors with less training learn how to recognize myeloma cells, the researchers also believe their dataset should be used to develop AI-based methods that can automatically differentiate plasma and non-plasma cells. This, they noted, would improve the diagnostic process for all clinicians and, in turn, for patients.
As a benchmark, the team used their dataset to train an AI-based algorithm to recognize plasma versus non-plasma cells in the bone marrow samples.
Overall, the model had good performance for correctly classifying the cells, the results showed. Across a group of 10 patients, the model-predicted disease status matched an expert’s diagnosis for nine of them.
We are confident that our dataset contains valuable patterns to identify plasma and non-plasma cells, providing an important and low-cost setup to support hematologists [experts in blood disorders].
“Considering all analyses … we are confident that our dataset contains valuable patterns to identify plasma and non-plasma cells, providing an important and low-cost setup to support hematologists,” the researchers wrote.
Given the simple, smartphone-based approach, the scientists emphasized that it can be made broadly accessible even in areas that are more resources-limited.
The team hopes that by making the dataset widely available, other scientists will build on their work to develop even better AI models for improving myeloma diagnosis.
“The availability of our dataset and benchmark model support ongoing research and development in the field, promoting continuous improvement in the accuracy and efficiency of MM diagnostics,” the team wrote.